Why Do We Need a Real Estate Document Management System?
Comments Off on Why Do We Need a Real Estate Document Management System?When it comes to real estate, documents are everywhere—from lease agreements and NOCs to inspection reports, client IDs, and purchase contracts. On average, real estate professionals spend about 50% of their time just preparing documents. Without a reliable solution, 25% of these documents are likely to get lost. Managing all this across departments, projects, and stakeholders is no small task.
In 2024, the U.S. real estate industry was valued at $236.4 billion. In a market this huge, how you manage your documents can make a big difference. That’s where a real estate document management system comes in. It helps property managers serve tenants and owners better, stay compliant, and quickly find any document—contracts, leases, or receipts—anytime, anywhere, with just a click.
Let’s explore how a real estate document management system can transform the way property managers securely handle the mountain of paperwork in both residential and commercial real estate.
Why Traditional Document Handling Fails in Real Estate
Some real estate companies still rely on manual processes, email threads, shared drives, or paper records. While this might work for smaller teams, it quickly becomes challenging for companies managing hundreds or thousands of units. It works… until it really doesn’t.
Let’s say, a leasing officer at a major developer is tasked with renewing a tenant’s expired contract across three inboxes and a USB from 2019. She finds it—five days too late. The result? An upset tenant, delayed renewal, and a not-so-happy line manager.
What is a Real Estate Document Management System?
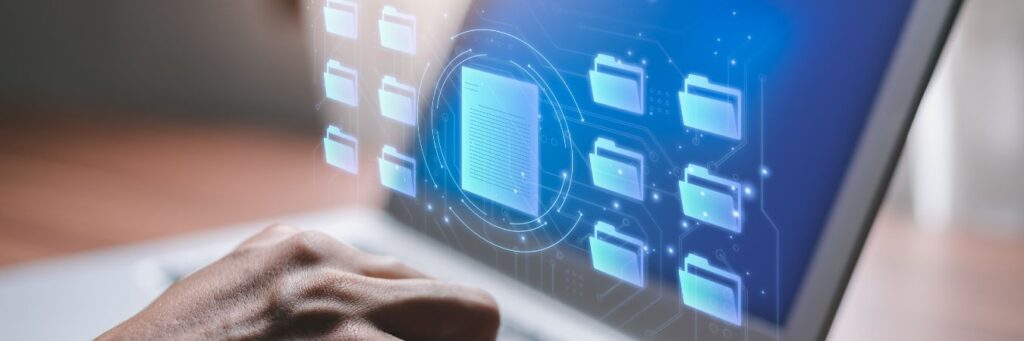
A Real Estate Document Management System is a specialized software that helps property developers, leasing teams, and facility managers store, manage, retrieve, and track documents related to customers, properties, and transactions—all from one centralized platform.
Think of it as the management team of your real estate business—keeping every agreement, ID proof, approval, and financial document not just stored but accessible, actionable, and secure.
Less paper shuffling, more deal closing.
Choosing the Right Document Management System
When evaluating a Real Estate Document Management System, ask:
- Can it handle property-specific document types like lease agreements and inspection reports?
- Does it offer expiration tracking and automated reminders?
- Is it integrated with the tools we already use for communications and collaborations?
- Does it offer secure, role-based access?
- Can it scale across multiple developments or regions?
Who Needs a Real Estate Document Management System?
If you’re in any of the following roles, this is for you:
- CEOs and CIOs looking to future-proof operations and cut down manual inefficiencies
- CFOs and Finance teams needing fast access to invoices, receipts, and compliance paperwork
- Leasing and Sales Heads handling multiple deals and customer interactions daily
- IT Managers looking for integrated and secure systems
- Operations Directors streamlining multi-property management
Benefits of Using a Real Estate Document Management System
1. All Your Docs, All in One Place
Say goodbye to scattered folders and endless email threads. With a centralized document repository, your sales, leasing, and legal teams can access documents from any project, anytime—from lease agreements and title deeds to NOCs and inspection reports—with just a few clicks in a single platform.
Imagine this: John is drafting a lease for a new tenant. At the same time, the legal team needs the title deed, and the sales team wants to check last year’s payment plan. Instead of chasing each other, they simply log into the real estate document management system, pull up the project, and access the files they need—instantly, from anywhere. No delays and no miscommunication, just seamless collaboration.
2. Track Expiry Dates Like a Pro
Staying on top of document expiry dates is critical. Whether it’s tenancy contracts, property management agreements, leasing licenses, or government approvals, missing a renewal can lead to legal trouble, fines, or even lost revenue.
A Real Estate Document Management System takes the stress out of this by automatically tracking expiry dates and sending timely alerts—well before deadlines approach.
Let’s say you’re managing multiple residential and commercial towers. Without a system, you have to track the expiries manually. But with a document management system in place, your dashboard shows upcoming expiries.
Like, “5 contracts in Tower A and 3 contracts in Tower C are expiring in the next 30 days.”
You get enough time to talk to tenants, update terms, or prep units for re-listing. This helps you retain tenants and avoid vacancies.
3. Stay Compliant with Custom Checklists
Every deal comes with its own set of documentation, especially when working with individual buyers, corporate tenants, or international investors. A document management system lets you create custom checklists for each type of client or transaction. Think items like passports, visas, trade licenses, and more.
Having a checklist means nothing gets missed, and the process stays fast, organized, and compliant.
4. Enable Seamless Team Collaboration
Real estate operations involve multiple departments—leasing agents, finance, legal, and senior management—all needing access to the same documents at different stages of a deal. A document management system allows everyone to collaborate in real time, even if they’re working from different locations.
Team members can add notes, give feedback, or approve documents without sending files back and forth or waiting for someone to be in the office. You could even integrate tools like SharePoint to create a common platform where everyone—from site offices to HQ—stays in sync.
Imagine a leasing agent finalizing a lease for a retail space and uploading the agreement into the system. The finance team reviews and adds a note on payment terms. The legal team checks compliance and flags a clause for revision. Meanwhile, the leasing manager approves the deal directly from an email notification— all within a few hours.
This kind of real-time collaboration speeds up the lease process and keeps everyone aligned—no delays, no miscommunication.
5. Secure and Role-Based Access
In real estate, your documents often include sensitive information—tenant IDs, payment details, legal agreements, and more. A document management system with role-based access controls ensures that only authorized team members can view or edit specific documents. This keeps your data secure and aligns with internal compliance policies.
Let’s say your leasing team needs access to signed lease agreements but not to the tenant’s payment history. Meanwhile, the finance team needs to view invoices and receipts, but shouldn’t access the tenant’s ID or passport copy. With role-based access, you can set permissions so each team only sees what’s relevant to them.
This helps protect confidential data, reduces the risk of errors, and ensures compliance with privacy policies and audit requirements.
6. E-Signatures and Faster Turnaround
Real estate deals often get delayed because of paperwork—especially when tenants, buyers, or signatories are in different locations. With e-signature tools like DocuSign, you can send lease agreements, sale contracts, or NDAs digitally and get them signed remotely in minutes.
Once signed, the document is automatically saved under the respective client or property record—no need to print, scan, or courier anything.
Operational Real Estate CRM+ Document Management System: Property-xRM
A Real Estate Document Management System isn’t just about staying organized—it’s about building a more responsive, compliant, and scalable operation. Whether it’s digitizing client onboarding, managing expiring contracts, or enabling seamless collaboration, the right system transforms how you work.
That’s where Property-xRM comes in. As an operational real estate CRM powered by Microsoft Dynamics 365, Property-xRM allows you to generate and manage documents —such as SPAs, Reservation Agreements, Invoices, and Receipts—enforce template standards, and securely store them—all within the CRM itself for quick and easy access.
Need renewal alerts or compliance notifications? Those can be triggered automatically from within the system too.
With built-in integrations to SharePoint, Outlook, and Teams, Property-xRM becomes more than just a document hub—it’s a centralized digital workspace for your sales, leasing, and operations teams.
If you’re looking to take control of document management and improve overall efficiency, Property-xRM is a solution worth exploring.
